Premium Only Content
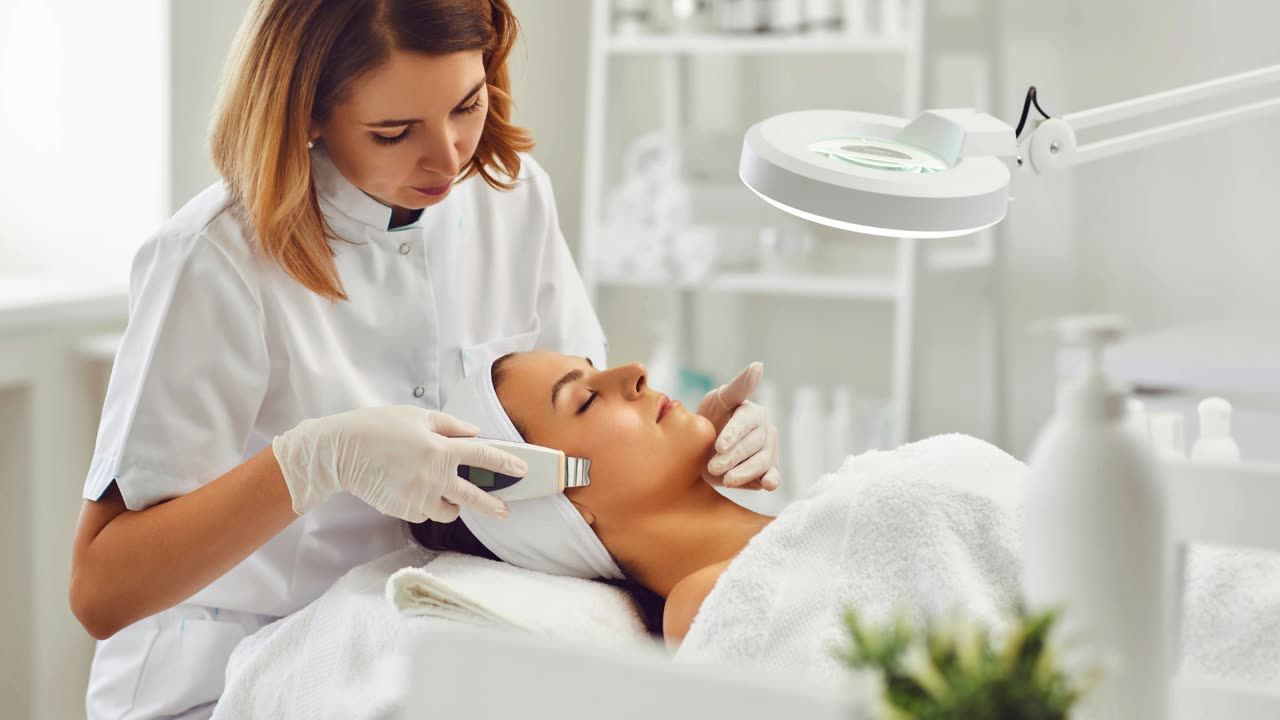
Artificial Intelligence or AI in Clinical Applications - Part 1
Cardiovascular:
Artificial intelligence algorithms have shown promising results in accurately diagnosing and risk stratifying patients with concern for coronary artery disease, showing potential as an initial triage tool. Other algorithms have been used in predicting patient mortality, medication effects, and adverse events following treatment for acute coronary syndrome. Wearables, smartphones, and internet-based technologies have also shown the ability to monitor patients' cardiac data points, expanding the amount of data and the various settings AI models can use and potentially enabling earlier detection of cardiac events occurring outside of the hospital. Another growing area of research is the utility of AI in classifying heart sounds and diagnosing valvular disease. Challenges of AI in cardiovascular medicine have included the limited data available to train machine learning models, such as limited data on social determinants of health as they pertain to cardiovascular disease.
A key limitation in early studies evaluating AI were omissions of data comparing algorithmic performance to humans. Examples of studies which assess AI performance relative to physicians includes how AI is non-inferior to humans in interpretation of cardiac echocardiograms and that AI can diagnose heart attack better than human physicians in the emergency setting, reducing both low-value testing and missed diagnoses.
In cardiovascular tissue engineering and organoid studies, AI is increasingly used to analyze microscopy images, and integrate electrophysiological read outs.
Dermatology:
Medical imaging (such as X-ray and photography) is a commonly used tool in dermatology and the development of deep learning has been strongly tied to image processing. Therefore, there is a natural fit between the dermatology and deep learning. Machine learning learning holds great potential to process these images for better diagnoses. Han et al. showed keratinocytic skin cancer detection from face photographs. Esteva et al. demonstrated dermatologist-level classification of skin cancer from lesion images. Noyan et al. demonstrated a convolutional neural network that achieved 94% accuracy at identifying skin cells from microscopic Tzanck smear images. A concern raised with this work is that it has not engaged with disparities related to skin color or differential treatment of patients with non-white skin tones.
Life Goals Achievement Success Guides. As well as helping you defeat: Lack of focus | Fear of failure | Lack of commitment | Lack of a plan | Procrastination | Lack of confidence | Analysis paralysis | Unrealistic goals | Lack of motivation. So you can live the life you love and love the life you live.
Visit us - https://breakouttools.com
-
LIVE
Caleb Hammer
13 hours agoShe’s Back | Financial Audit
135 watching -
LIVE
Wendy Bell Radio
6 hours agoAmerica's Arsonist
11,609 watching -
LIVE
Barry Cunningham
4 hours agoWATCH LIVE BREAKING NEWS: PRESIDENT TRUMP GETS SENTENCED!
891 watching -
LIVE
Pepkilla
1 hour agoGaming Limbo ~
197 watching -
15:27
Dave Portnoy
2 hours agoDavey Day Trader Presented by Kraken - January 10, 2025
8.98K4 -
2:45:56
Matt Kohrs
14 hours agoUnemployment Report, Bitcoin Tilted & Breaking Tesla News || The MK Show
27.8K4 -
34:50
BonginoReport
5 hours agoClimate Cult Has No One To Blame But Itself (Ep.116) - 01/10/2025
56.9K71 -
LIVE
Vigilant News Network
15 hours agoFlorida Grand Jury Drops Bombshell on COVID Jab Manufacturers | The Daily Dose
820 watching -
1:45:54
Jeff Ahern
2 hours ago $1.51 earnedFriday Freak out with Jeff Ahern (Nobody likes Kamala and the LA Fires)
8.83K -
1:06:47
Game On!
9 hours ago $2.05 earnedThere's NO WAY Ohio St vs Texas can live up to the HYPE!
20.6K3