Premium Only Content
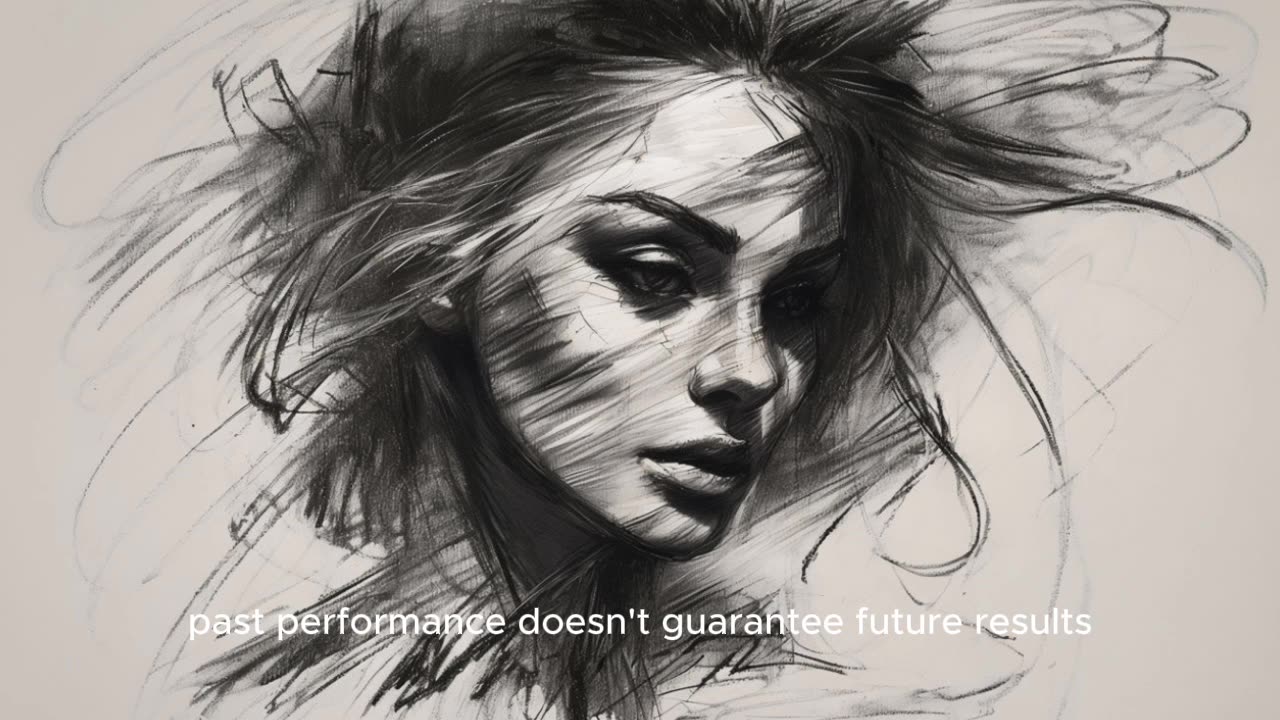
Online Learning Techniques for Prediction of Temporal Tabular Datasets with Regime Changes
This discussion examines the challenges of applying machine learning techniques to non-stationary temporal datasets, particularly financial data, which exhibit regime changes and high stochasticity. The authors propose a modular machine learning pipeline for ranking predictions on temporal panel datasets, emphasising robustness under regime changes. The pipeline utilises gradient boosting decision trees, outperforming neural network models in terms of generalisability and computational efficiency. The paper further demonstrates how online learning techniques can be employed to enhance predictions, particularly dynamic feature projection for improving robustness and dynamical model ensembling for enhancing Sharpe and Calmar ratios. The authors meticulously address issues of data leakage and model reproducibility, contributing to a more reliable and transparent approach for financial prediction.
Link to the paper: https://arxiv.org/pdf/2301.00790
Nosacapital Square (On Binance): https://www.binance.com/en/square/profile/NosaCapital
Open a Free Morpher Account (and get free tokens): https://nosax.me/morpher
#nosacapital #machinelearningmodels #machinelearning #machinelearningalgorithm #AI #aipodcast #finance #quantitativetrading #calmarratios #machinelearningensembling
-
LIVE
The Big Migâ„¢
16 hours agoGlobal Finance Forum Powered By Genesis Gold Group
88 watching -
LIVE
Caleb Hammer
2 hours agoEgo-Maniac Thinks She Can Manifest Problems Away | Financial Audit
325 watching -
LIVE
LFA TV
5 hours agoSPEAKER VOTE LIVE! | LIVE FROM AMERICA 1.3.25
6,490 watching -
LIVE
SpartakusLIVE
3 hours agoDelta Force w/ Phixate || Lord of the Loot secures the JUICE
225 watching -
16:49
Dave Portnoy
3 hours agoDavey Day Trader Presented by Kraken - January 3, 2025
25.5K3 -
2:16:11
Matt Kohrs
8 hours agoTesla Falls, Nvidia Bounces & Payday Friday || The MK Show
35K5 -
1:33:19
Tactical Advisor
3 hours agoThe Vault Room Podcast 007 | Terrorist Attacks Update
24.8K1 -
1:45:30
Game On!
12 hours ago $1.58 earnedNotre Dame proves that the SEC is the WORST conference in college football!
18.9K5 -
1:49:52
Jeff Ahern
3 hours ago $2.47 earnedFriday Freak out with Jeff Ahern ( The Dr Michael Schwartz interview)
26.9K2 -
15:04
Misha Petrov
22 hours agoThese Leftist Tattoos Are UNHINGED!
34K82