Premium Only Content
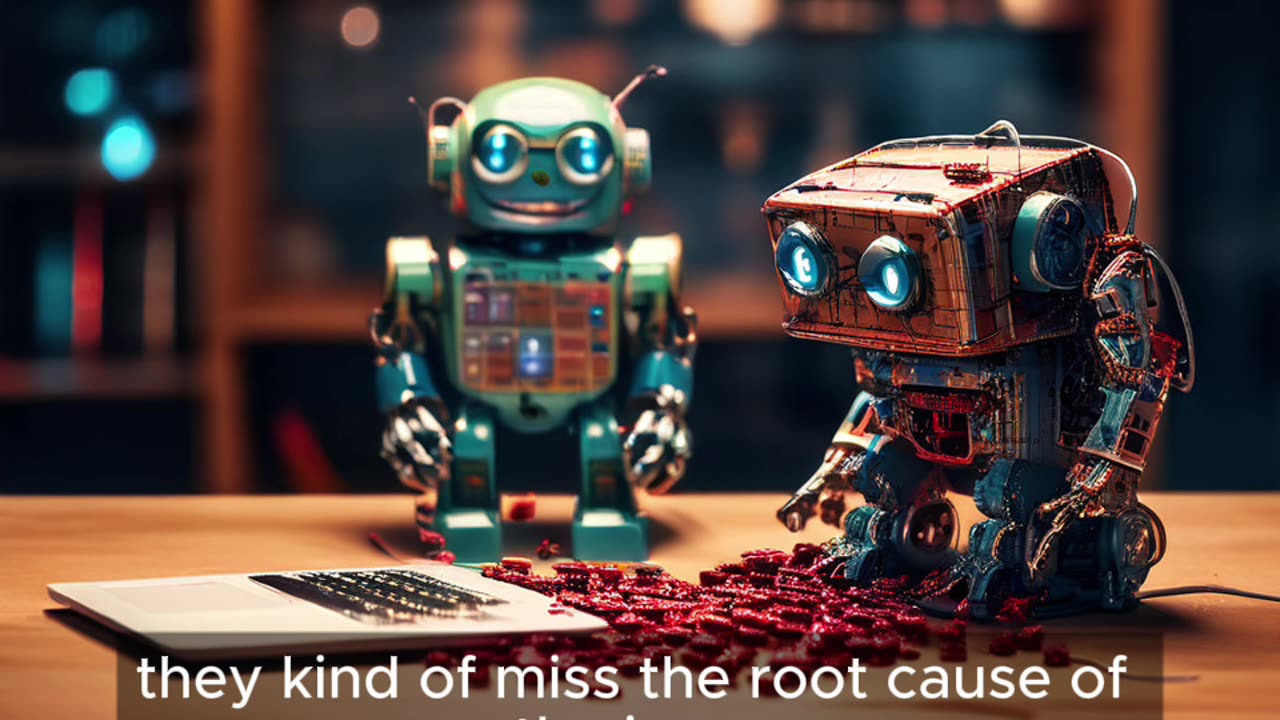
Improving Generalization Performance by Switching from Adam to SGD
This paper investigates a method called SWATS, which switches from an adaptive optimization method like Adam to SGD (Stochastic Gradient Descent) during training. This approach aims to combine the benefits of both optimizers, namely, Adam's fast initial progress and SGD's superior generalization performance. The paper proposes a criterion for determining the optimal switch point and uses a projection-based method to estimate the learning rate for SGD after the switch. The effectiveness of SWATS is demonstrated through experiments on various image classification and language modeling tasks, showing comparable performance to the best individual optimizer.
The paper can be found here: https://www.semanticscholar.org/reader/8f253d759d99e92888bf9eb595c59cf962fd9069
All Images by NosazenaNFT: https://linktr.ee/nosazenanfts
Nosacapital Square (On Binance): https://www.binance.com/en/square/profile/NosaCapital
Open a Free Morpher Account (and get free tokens): https://nosax.me/morpher
#nosacapital #machinelearning #machinelearningmodels #SGD #ADAM #SWATS
-
1:03:34
In The Litter Box w/ Jewels & Catturd
23 hours agoCONFIRM KASH NOW! | In the Litter Box w/ Jewels & Catturd – Ep. 742 – 2/14/2025
43.3K27 -
13:38
Simply Bitcoin
10 hours ago $1.70 earnedFED’s Worst NIGHTMARE Just Got WORSE | $13M Bitcoin is Coming!
48.2K3 -
10:25
Megyn Kelly
1 day agoNew Video and Details Emerge Showing "Serial Fraudster" Blake Lively's Past Actions, with Ruthless
33.7K12 -
1:21:04
Twins Pod
15 hours agoElon Took Away His X Verification, I Wonder Why... | Twins Pod - Episode 52 - Jake Shields
54.9K8 -
6:01:44
bltgamers
7 hours ago🟢LIVE -Tomb Raider Adventure on Valentine's Day
16.3K2 -
LIVE
John Crump Live
21 hours agoLive Call In Show!!!
68 watching -
1:38:12
The Officer Tatum
4 hours agoLIVE Security Guard POWER SLAPS Black Woman as LEFTISTS Cry OVER RFK Jr. + More Ep 62
37.4K39 -
1:46:13
The Quartering
7 hours agoDOJ Employees QUIT, Mass Federal Layoffs Start, and Kanye Divorce Rumors
78.1K34 -
1:01:13
Dr. Eric Berg
4 days agoThe Dr. Berg Show LIVE February 14, 2025
37K4 -
40:17
SLS - Street League Skateboarding
2 days agoEVERY 9 CLUB IN FLORIDA! Looking back at SLS Jacksonville 2021 & 2022 - Yuto, Jagger, Sora & more...
23.7K1