Premium Only Content
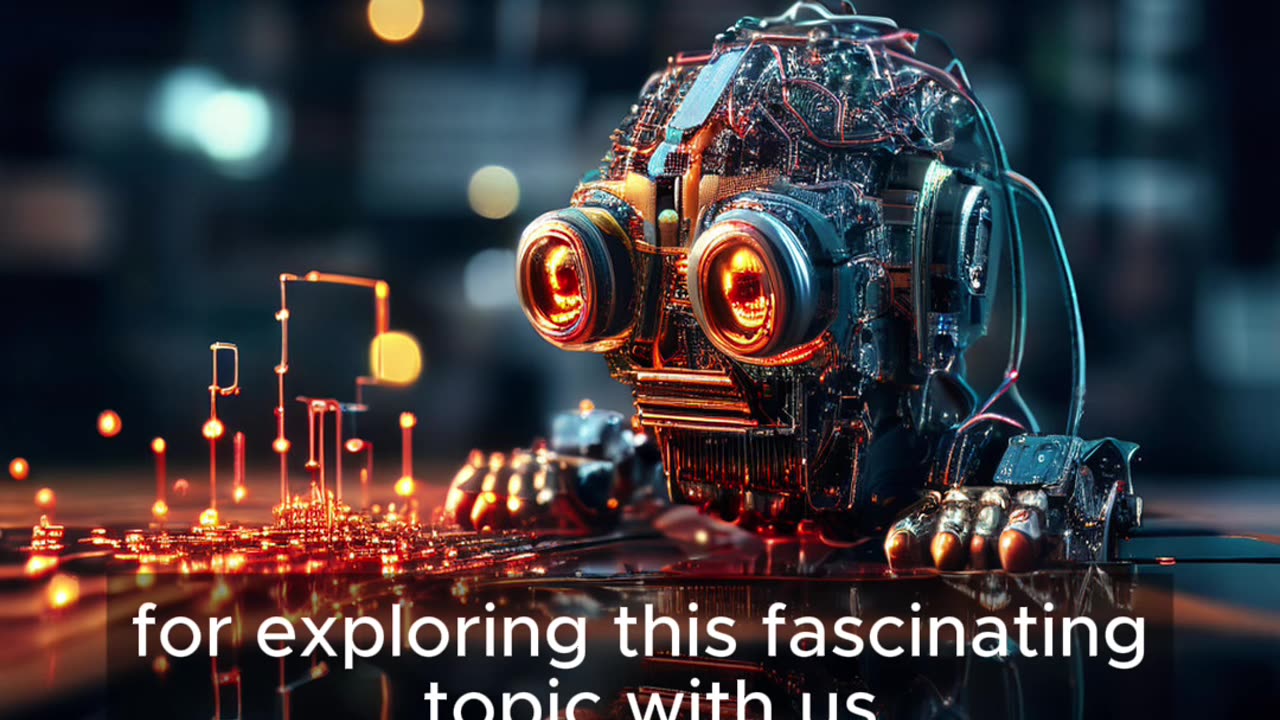
Ensemble Selection from Libraries of Models
This paper describes a method for building ensembles of machine learning models called ensemble selection, which uses a forward stepwise selection process to identify the best subset of models from a large library of models trained with different algorithms and parameters. The authors demonstrate that ensemble selection outperforms other ensemble methods, such as bagging and boosting, on a variety of metrics and data sets. The effectiveness of ensemble selection is attributed to its ability to optimise ensembles to specific performance metrics while mitigating overfitting through techniques like bagged ensemble selection and sorted ensemble initialisation.
Find the paper: https://www.cs.cornell.edu/~alexn/papers/shotgun.icml04.revised.rev2.pdf
All Images by NosazenaNFT: https://linktr.ee/nosazenanfts
Nosacapital Square (On Binance): https://www.binance.com/en/square/profile/NosaCapital
Open a Free Morpher Account (and get free tokens): https://nosax.me/morpher
#nosacapital #machinelearning #machinelearningmodels #ensemble
-
18:37
SantaSurfing
8 hours ago4/17/2025 - Trouble for Tish/Google/Jerome! Trump stops a war!
18.6K15 -
49:39
Man in America
12 hours agoThey’re Feeding Us POISON and Calling It Dinner w/ Kim Bright
33K10 -
LIVE
BrancoFXDC
2 hours ago $1.25 earnedWarzone Rounds - DAY 10 of no Internet
205 watching -
1:17:17
RiftTV/Slightly Offensive
7 hours ago $6.62 earnedMASSACRE at FSU: Who was Actually RESPONSIBLE? | Slightly Offensive
30.7K12 -
SilverFox
3 hours ago🔴LIVE - HUGE UPDATE! LORDS OF THE FALLEN 2.0
19.1K -
2:03:46
Roseanne Barr
7 hours ago $19.17 earned"God, Go Get em' Honey" W/ Tal Oran | The Roseanne Barr Podcast #95
99.6K41 -
5:53:59
BSparksGaming
6 hours agoLords of the Fallen Version 2.0 Gameplay!
13.9K -
1:04:45
Donald Trump Jr.
9 hours agoThe Left’s Lunacy Knows No Bounds, Plus Taking Your Questions Live! | Triggered Ep234
140K154 -
4:05:32
Rebel News
13 hours ago $16.82 earnedLIVE: Rebels react as leaders face off in Day 2 English debate
91.8K43 -
LIVE
Phyxicx
7 hours agoMore Halo I guess - 4/17/2025
110 watching