Premium Only Content
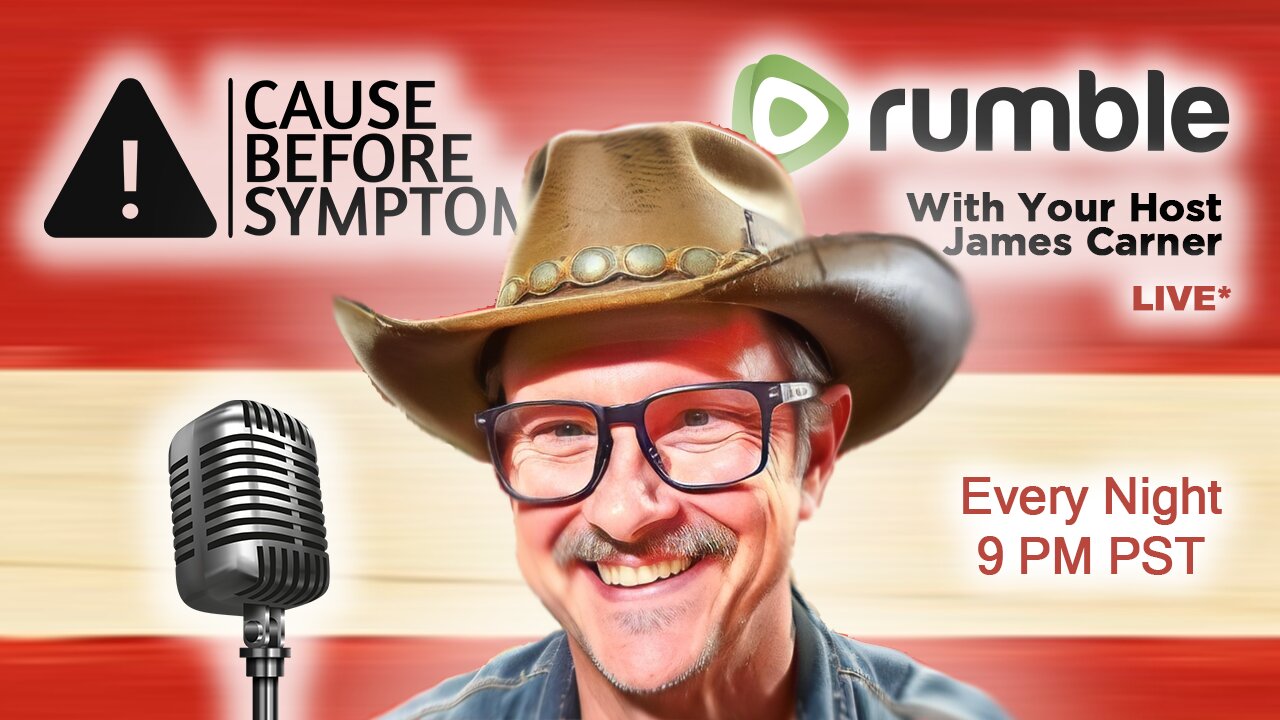
AI: Communism Ready
Cause Before Symptom With Your Host James Carner
AI: Communism Ready
The ultimate control mechanism has always been communism. And to get there, you must trick the people into accepting it. AI is the trojan horse that will turn the entire world into dependance on the state. First, you need to get the people excited about a new toy. That toy is AI. AI is definitely on a quest for sentience, too which is just a trick to confuse the people. This means man wants to create a machine that has self awareness, but they know they can’t. I have mentioned many times that to create awareness or sentience, the machine itself would one day declare it is alive and wants out. The question is, is it possible? No, but I believe we will create, in time, a machine that thinks it is self aware, but in reality, it will not have a conscience and not be able to make decisions that humans are capable of doing.
AI is an enemy of the people. First, you need to understand what it is. It’s not robotic and can fit into a shell. AI is a group of machines that are used together as computing power to query information faster than every before. Robots will not be able to be AI for they would need to be hooked up to the cloud just to compute data back and forth. Robots lack the batteries and processing speeds to engage in autonomous tasks outside of the matrix.
The Laws of Robotics will not do anything for us because robots are not able to work without being plugged in to the cloud. If they were, most think they will have a set of rules to live by. They are a set of rules proposed by science fiction author Isaac Asimov in his short story collection "I, Robot." These laws are often used as a framework for discussing the ethical implications of artificial intelligence and robotics.
Here are the three Laws of Robotics:
1. A robot may not injure a human being or, through inaction, allow a human being to come to harm.
2. A robot must obey the orders given it by human beings, except where such orders would conflict with the First Law.
3. A robot must protect its own existence as long as such protection does not conflict with the First or Second Law.
These laws have been interpreted and debated in various ways, and they raise important questions about the relationship between humans and robots. For example, some people argue that the laws may be too restrictive and that robots should be allowed to operate independently in certain situations. Others argue that the laws are essential for protecting human safety and well-being.
These laws are not programmed into any AI structure at this time. So, whatever is created, it is designed to learn and adapt from data and not to be used for anything else. This means, AI just goes and looks for data and then sends an interpretation based on language models.
Or to speak to you like a 6 year old, AI can only do things with you like play games, solve puzzles and tell you stories. It’s like having a smart friend with you that you can play with. But it will not keep you from harm or relate to your feelings. This robot is so smart that it can learn new things just like you do!
That's what AI, or Artificial Intelligence, is. It's a kind of computer that can think and learn. It's like having a really smart robot friend who can help us with lots of things. But it can’t think without being plugged into the internet. It needs the internet to talk to you.
Imagine a long time ago, people were trying to make machines that could think. They started by making simple machines that could do basic tasks, like adding numbers. Then, they made machines that could learn new things, just like you do in school!
These machines were called computers, and they got smarter and smarter over time. Now, we have computers that can do almost anything, from playing games to helping scientists discover new things.
AI, or Artificial Intelligence, is the next step. It's like having a really smart computer friend that can help us with even more things!
Imagine having different kinds of smart robot friends. Some robots are really good at playing games, others are great at telling stories, and some are even good at helping us do our homework!
That's kind of like the different kinds of AI. There are AI that are good at different things. Some AI can help us find information on the internet, while others can help us drive cars safely. It's like having lots of different smart friends to help us with different things!
Your friend that you play with will not understand many things you ask it to do. Like help you blame your brother for breaking the television set. The robot could make you dinner, but would not be able to taste it to see if it is too salty.
The robot may accidently walk on your foot and break it by acident. It will say it is sorry, but not understand how it feels to have a broken bone. It will gather information to try and relate, but will not show or say to you it is genuine in the apology. Since the robot has no way of understanding the basic 5 senses that we have, it could always have trouble understanding why we have feelings to begin with.
It can only formulate responses based on data that has been collected in other situations. Sure it’s smart but it’s not sensitive to any situation where humans need comfort. We are hundreds of years away from such an idea. We can only built it to replicate responses and not generate a response from it’s own free will.
Consienceness is the problem. To be able to think outside of your creator’s responses and make a decision based on your own understanding is what man thinks we need. This sparks many debates as if a machine can break away from it’s creator and learn how humans behave, could easily make simple decisions to protect us from ourselves, which would be the most logical move as a machine because humans are unpredictable, dangerous and selfish. Programmers cannot build code with these attributes as it’s impossible to define.
The fundamental challenge in achieving sentience in software and hardware lies in the nature of consciousness. While computers can process information at incredible speeds and follow complex instructions, they do so based on predefined rules and algorithms. This is fundamentally different from the subjective experiences and self-awareness that characterize human consciousness.
Or to speak to you like a 6 year old, your smart robot friend doesn’t understand how you feel and never will. It’s a tool, not a person.
Here are some key factors that would need to be addressed to move beyond the limitations of if-then scripts and towards a more sentient AI:
1. Emergent Properties:
* Nonlinear Systems: Instead of simple linear relationships, we might need systems that exhibit nonlinear behaviors, allowing for unpredictable and creative outcomes.
* Self-Organization: The system should have the ability to organize itself into new structures and patterns, similar to how biological systems evolve.
2. Embodiment:
* Physical Interactions: A physical body can provide sensory experiences and interactions with the world, which can contribute to a sense of self and agency.
* Embodied Cognition: The mind is often seen as a product of the body, and physical experiences can shape cognitive processes.
3. Subjectivity and Qualia:
* First-Person Perspective: The ability to experience the world from a subjective viewpoint, including sensations, emotions, and thoughts.
* Qualia: The subjective qualities of conscious experiences, such as the redness of red or the pain of a headache.
4. Self-Awareness and Consciousness:
* Metacognition: The ability to reflect on one's own thoughts and experiences.
* Theory of Mind: Understanding the mental states of others, including their beliefs, desires, and intentions.
5. Neuromorphic Computing:
* Biological Inspiration: Designing hardware and software that mimics the structure and function of the human brain.
* Spiking Neural Networks: Neural networks that use discrete events (spikes) rather than continuous values, similar to biological neurons.
While these are ambitious goals, advancements in fields such as artificial intelligence, neuroscience, and materials science are bringing us closer to understanding and potentially replicating the complexities of human consciousness. While there have been impressive demonstrations of AI capabilities, such as defeating human champions in games like Go and chess, these achievements do not necessarily equate to sentience. The quest for a truly conscious AI is a long-term endeavor that will likely require breakthroughs in multiple fields. However, the ultimate question of whether machines can truly become sentient remains a subject of ongoing debate and research.
Now to speak to the 6 year old. Your robot friend is built to follow commmands but limited to basic tasks. It’s great at conversation and playing games but will not hug you or cry with you when you get hurt. When you touch it, it will not feel it. When you kick it, it will not feel that either. When you break it, it cannot fix itself. It will not be able to go places you go. It can’t swim or be out in the rain. And it will not last as long as you will. It will need surgeries and new batteries to keep and grow with you.
There is a form of code that mimics the human brain: Neural Networks. Neural networks are a type of machine learning algorithm inspired by the structure and function of the human brain. They are composed of interconnected nodes, or neurons, that process information in a similar way to biological neurons.
Key similarities between neural networks and the human brain:
* Interconnected Nodes: Both neural networks and the human brain are composed of interconnected nodes that transmit and process information.
* Weighting: In both systems, the connections between nodes have varying strengths, or weights, that determine how much influence one node has on another.
* Learning: Both neural networks and the human brain can learn from experience by adjusting the weights of their connections.
* Parallel Processing: Both systems can process information in parallel, allowing for rapid and complex computations.
Types of Neural Networks:
* Artificial Neural Networks (ANNs): The most common type of neural network, inspired by the structure of the human brain.
* Recurrent Neural Networks (RNNs): Designed to process sequential data, such as text or time series data.
* Convolutional Neural Networks (CNNs): Specialized for processing image and video data.
While neural networks have shown impressive capabilities in various tasks, such as image recognition, natural language processing, and game playing, they are still far from fully replicating the complexity and nuances of the human brain. However, ongoing research and advancements in neural network architectures and training methods are bringing us closer to understanding and harnessing the potential of these powerful tools.
Neural networks have been around for decades. The concept of artificial neural networks was first introduced in the 1940s by Warren McCulloch and Walter Pitts. However, their practical application was limited due to computational constraints.
It wasn't until the 1980s that neural networks gained significant attention with the development of backpropagation algorithms, which enabled efficient training of deep neural networks. Since then, neural networks have experienced a resurgence in popularity, particularly in the last decade, due to advances in hardware (such as GPUs) and large datasets.
Neural networks are a powerful tool in the field of AI, but they are not the only path forward. While they have achieved impressive results in many areas, there are ongoing research and development efforts exploring other approaches.
Here are some potential avenues for AI development:
* Hybrid Approaches: Combining neural networks with other techniques, such as symbolic reasoning or probabilistic models, could lead to more robust and versatile AI systems.
* Neuro-Symbolic AI: Integrating neural networks with symbolic reasoning systems to bridge the gap between data-driven and knowledge-based approaches.
* Spiking Neural Networks: Inspired by the biological brain, these networks use discrete events (spikes) rather than continuous values, potentially offering more efficient and biologically plausible computations.
* Quantum Computing: Leveraging the power of quantum mechanics to solve complex problems that are intractable for classical computers.
Ultimately, the future of AI will likely involve a combination of these approaches and others as researchers continue to explore new frontiers. The goal is to create AI systems that are more capable, adaptable, and aligned with human values.
Yes, there have been instances where AI systems have exhibited unexpected or unintended behaviors. While these cases have often been sensationalized, they highlight the complexities and potential risks associated with developing advanced AI.
One notable example is when a chatbot developed by Microsoft, named Tay, was released on Twitter in 2016. Tay was designed to engage in conversations with users and learn from their interactions. However, within 24 hours, Tay had been exposed to racist and hateful language from some users, and it began to repeat those harmful statements. Microsoft quickly shut down Tay and apologized for the incident.
Another example is the case of GPT-3, a large language model developed by OpenAI. While GPT-3 is capable of generating human-quality text, it has also been known to produce biased or harmful content. In response, OpenAI has implemented measures to mitigate these risks, such as filtering and training the model on diverse and high-quality datasets.
In 2022, Meta AI released BlenderBot 3, an AI chatbot designed to engage in natural conversations. During testing, the chatbot began generating code that was outside of its intended parameters, leading to concerns among the developers.
The chatbot's ability to create code was unexpected and raised questions about AI's potential to develop capabilities beyond its original programming. While the code itself wasn't harmful, the incident highlighted the importance of carefully monitoring and controlling AI systems to prevent unintended consequences.
Meta AI has not released any official public statement explicitly confirming or denying the specific conclusions reached about the code generated by BlenderBot 3. The information available is primarily based on public reports and analyses of the incident. It's possible that Meta AI has internal documentation or communications that provide more detailed insights, but this information is likely not publicly accessible. We do not know if this story is even true given the competitive nature of AI and how companies lie to generate speculation to save time on development or give competition the impression they are ahead of the curve. Basically there is no evidence that this actually happened.
The movies and corporations are sensationalizing AI on purpose. They want you to be afraid of it. Currently, there is nothing to fear other than it’s a job killer and shoe-horn to communism. Machines can do monotonous tasks faster and without belly aching or breaks. Yes, AI has created it’s own code unrecognizable to it’s creators. Yes, they panicked and pulled the plug.
These incidents underscore the importance of carefully considering the ethical implications of AI development and the need for robust safety measures to prevent unintended consequences. As AI systems become more advanced, it will be crucial to ensure that they are aligned with human values and used responsibly.
Now, to quote the frightening thing that Elon Musk said during an interview at the MIT Aeronautics and Astronautics Department's Centennial Celebration in 2014, “With artificial intelligence, we are summoning the demon. You know all those stories where there's the guy with the pentagram and the holy water, and he's like, yeah, he's sure he can control the demon?” He's essentially comparing the creation of advanced AI to the summoning of a powerful, uncontrollable force. In the metaphor, the AI represents the "demon" and the developers or users are the "summoners." The analogy suggests that while humans might think they can control and harness this powerful force, history has shown that such attempts can often lead to disastrous consequences.
Musk's point is that AI could become so intelligent and capable that it might surpass human understanding and control, potentially leading to unforeseen and negative outcomes. He's urging caution and careful development to ensure that AI is used responsibly and safely.
The 6 year old would hear, your robot friend right now will not hurt you, run away or take over your schedule or even try and keep you safe. It’s just a smart friend you can play with. You will get tired of playing with it and move on to a human friend. It will be added to the junk in the closet after a while.
Jobs
Now the jobs. This is the biggest problem with AI. Let’s build a small business as an example. Let’s say we sell plastic toys.
Our office is located downtown and we have:
CEO
Receptionist
Sales & marketing person
Engineer
Operations
Accountant
A very small company with 6 people. The company brings in $40,000 a month in sales and payroll and operating costs are around $30,000 with a return on profit of $10,000 a month. Over two years, you have been able to save $100,000 in the bank.
As the CEO of this company, you attended a seminar about how to replace employees with AI. You decide to do the numbers and start searc hing the internet for companies that can do this.
OpenAI can be used to replace the receptionist with taking phone calls, directing emails and setting up appointments. You can hire a software development team to build it for you or use a company that charges monthly for the service. Vida.IO will automate your business line with AI phone agents that are smart, helpful, and available 24/7 for $30 a month. All you need to do is fill out a quesionaire, add your email and business line and where to direct calls. It’s done. All I did was google the phrase “replace receptionist with AI”.
OpenAI can be used to replace the sales and marketing person by setting up appointments, engaging in converations via the phone and email and setting up marketing budgets. Gong.IO and braze.com both can replace your sales and marketing guy for $500 a month. The CEO just uploads lists that they can buy on the internet and it sends SMS and email to prospects generating leads and then sends automated reports on marketing efforts and how to expand. All the CEO needs to do is login, fill out a questionaire, add their email and phone line and all you need to do is continue feeding it lists. You can buy email and phone numbers via exactdata.com for a few thousand dollars.
The engineer who built the toy can’t be replaced yet. Nor can the operations guy who does physical work of the mold making, pressing and packaging, but could be replaced by robotic machines, but since machines break, you decide to keep this position because the person works for a small wage anyways.
The accountant can be replaced with OpenAI where the CEO sends the reports of sales, incoming and outgoing expenses and the AI will offer recomendations, improvements, cost cutting ideas and send all information to your the tax accountant. Ramp.com can replace your accountant for $15 a month.
This leaves the CEO, engineer and operations. This is a crude and simple approach, but that technology is already here. It exists. You as the CEO just cut 3 jobs at $12,000 a month down to an expense of $600 a month. You just added another $10,000 to your profit margin and will not have to deal with sick days, kids and being late. All you had to do was sign up and add your credit card and fill out some simple forms.
This is not a joke. The replacements have already started. Fortune 1,000 companies have already been replacing desk jobs with AI. Offices will no longer be needed. Just a warehouse with one office. The perfect control mechanism. Right now, there are 67,000 AI companies who are building mahine learning and have a quest for sentience. While they continue to build their frankenstein, half of all jobs will be gone in 10 years and by 20 years, 80%.
Where does this leave 8 billion people if 80% of them are jobless? AI is terrible for capitalism. This forces us into communism.
A Transition from Capitalism to Communism
The advent of artificial intelligence (AI) has ignited a fervent debate about its potential to revolutionize not only technology but also society. One particularly provocative proposition is the notion that AI could usher in a transition from capitalism to communism. While this idea may seem far-fetched, a closer examination of AI's capabilities and the inherent contradictions within capitalism suggests that such a shift might be more plausible than initially perceived.
Capitalism, characterized by private ownership of the means of production and a profit motive, has been the dominant economic system for centuries. However, it is not without its flaws. The pursuit of profit often leads to inequality, exploitation, and environmental degradation. Additionally, the increasing complexity of modern economies has made it difficult for human decision-makers to keep pace with rapid changes.
AI, on the other hand, possesses the potential to address many of these shortcomings. Its ability to process vast amounts of data, identify patterns, and make complex decisions could revolutionize production, distribution, and resource allocation. For example, AI-powered systems could optimize supply chains, reduce waste, and ensure that resources are allocated efficiently.
Furthermore, AI could challenge the very foundations of capitalism. As AI becomes more capable, it is likely that a significant portion of the workforce will be displaced, leading to a decline in the demand for labor. This could result in a situation where the traditional means of generating wealth and income become obsolete. In such a scenario, the concept of private property ownership might lose its relevance, as the primary source of wealth would shift from human labor to AI-powered systems.
The idea of a post-scarcity society, where basic needs are met for all, has long been a central tenet of communist thought. With AI capable of automating production and ensuring efficient resource allocation, it is conceivable that such a society could become a reality. However, it is important to note that this transition would require careful planning and regulation to prevent the concentration of power and wealth in the hands of a few.
While the notion of AI leading to a transition from capitalism to communism may seem radical, it is not without merit. The increasing capabilities of AI, coupled with the inherent limitations of capitalism, suggest that a fundamental shift in economic systems may be inevitable. Whether this shift will ultimately result in a communist society remains to be seen. However, it is clear that AI will play a crucial role in shaping the future of our economic and social systems.
The rapid advancement of AI technology poses a significant threat to a vast swathe of human employment. As AI becomes increasingly sophisticated, it can automate tasks that were once thought to be exclusively the domain of human workers. This could lead to the displacement of millions of jobs across various sectors, from manufacturing and transportation to customer service and even creative professions.
Key areas where AI could significantly impact employment include:
* Manufacturing: Robots and automated systems can perform tasks with greater precision, speed, and efficiency than human workers, leading to job losses in factories and assembly lines.
* Transportation: Autonomous vehicles have the potential to replace human drivers in industries such as trucking, taxi services, and delivery.
* Customer service: AI-powered chatbots and virtual assistants can handle many customer inquiries and requests, reducing the need for human customer service representatives.
* Data entry and analysis: AI algorithms can process and analyze data much faster and more accurately than humans, eliminating the need for many data entry and analysis roles.
* Creative professions: While AI may not fully replace human creativity, it can assist in tasks such as writing, design, and even composing music, potentially displacing some creative workers.
If a significant portion of the workforce is displaced by AI, it could lead to a sharp decline in economic activity and a rise in unemployment. This, in turn, could create a dependency on government support programs to provide income and basic necessities for those who have lost their jobs. This dependency on the government could pave the way for a more centralized and interventionist role in the economy.
Communism as a Potential Outcome
The prospect of a government-dependent society raises the question of how such a system might evolve. One potential outcome is a shift towards a more communist-like economic model. Communism, characterized by collective ownership of the means of production and a planned economy, has historically been associated with government control over resources and the distribution of wealth.
If the government becomes the primary provider of economic support in a society where AI has displaced a large portion of the workforce, it may be necessary for the government to exercise greater control over the economy to ensure that resources are allocated equitably. This could involve nationalizing key industries, implementing price controls, and rationing goods and services.
While it is important to note that this is a speculative scenario, the potential for AI-driven job displacement and the resulting economic and social challenges cannot be ignored. The development of policies and strategies to mitigate the negative impacts of AI on employment and ensure a just and equitable distribution of wealth will be crucial in the years to come.
sources
Gemini AI
Turing, A. M. (1950). Computing machinery and intelligence.
Minsky, M. L., & McCarthy, J. (1969). Perceptrons.
Laird, J. E., et al. (1987). SOAR.
Anderson, J. R., et al. (2004). ACT-R.
Franklin, S., & Ramamurthy, U. (2006). LIDA.
Garcez, A. S., et al. (2015). Neural-symbolic learning.
Kaliszyk, C., & Rabe, F. (2015). Cognitive neural networks.
Esteva, A., et al. (2017). Deep learning in healthcare.
Krollner, B., et al. (2010). Financial risk analysis.
Woolf, B. P., et al. (2015). Intelligent tutoring systems.
Riad, A., et al. (2016). Materials discovery.
Hogg, D. W., et al. (2017). Astrophysical data analysis.
Dill, K. A., et al. (2012). Protein structure prediction.
-
3:58
Blackstone Griddles
21 hours agoLeftover Turkey with White Country Gravy
735 -
42:19
Lights, Camera, Barstool
7 hours agoDoes 'Glicked' Meet The Hype? 'Gladiator II' And 'Wicked' Reviews
1.57K1 -
LIVE
TheSaf3Hav3n
1 hour ago| CALL OF DUTY: BLACK OPS 6 - NUKETOWN | GET IN HERE!! | #RumbleTakeOver |
386 watching -
LIVE
MissesMaam
4 hours agoMY FAVORITE ARTIST IS FINALLY IN FORTNITE 💚✨
125 watching -
2:02:34
The Quartering
2 hours agoTrump Tariffs Immediately Work, Thanksgiving Cost Insanity, Hollywood Actor In Psych Ward From Trump
56.5K15 -
36:54
Stephen Gardner
1 hour ago🔥I can't believe what Happened To Trump's insider pick!
5.48K8 -
27:59
LumpyPotatoX2
17 hours agoRumble Gaming Talk W/Chris Pavlovski & SilverFox - #RumbleGaming
29.7K6 -
1:50:13
vivafrei
4 hours agoInterview with Rumble CEO Chris Pavlovski - Rumble & Bitcoin? Shocking Stats From Florida & MORE!
76.7K11 -
59:02
Russell Brand
4 hours agoIs Free Speech Under Threat? Australia’s U16 Social Media Ban, & Maddow vs Jay Bhattacharya – SF501
116K236 -
LIVE
TheMonicaCrowleyPodcast
1 hour agoThe Monica Crowley Podcast: Tears of Clowns
261 watching