Premium Only Content
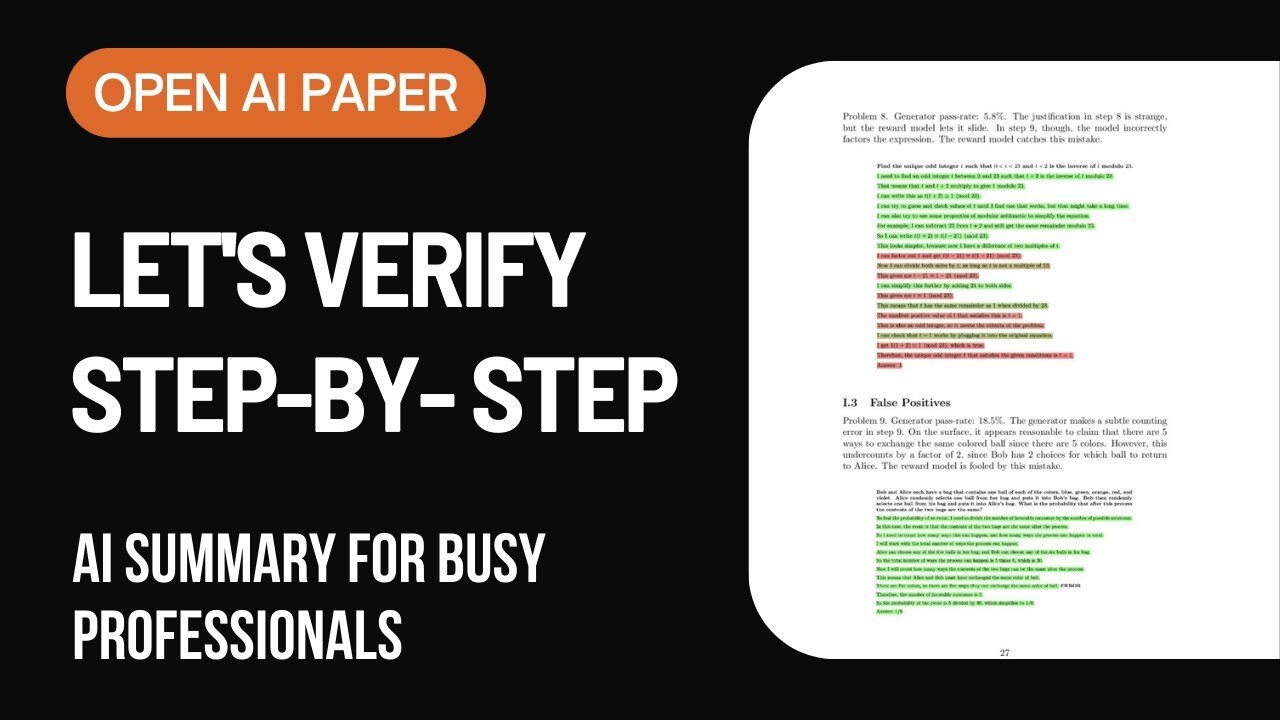
AI Summary - Let's Verify Step By Step - Improving Language Model Reasoning with Process Supervision
In this comprehensive video, we delve into the fascinating world of artificial intelligence, focusing specifically on the research paper "Step by Step" published by OpenAI. This video aims to unpack the complex concepts laid out by the authors and discuss their remarkable findings. This description will provide a concise summary of the key points covered.
🕒 TIMESTAMPS 🕒
00:00 Introduction
00:45 Paper Overview
01:19 Process Supervision vs. Outcome Supervision
01:45 Synthetic Supervision & Active Learning
02:21 Discussion of Related Work
02:49 Active Learning and Iterative Retraining
03:27 Evaluation on AP Exams and AMC Tests
03:57 Advantage of Process Supervision
04:21 Visualization of PRM's Performance
04:45 False Positives Analysis
05:12 Examples of Mistakes in Mathematical Reasoning
05:36 Direct Comparison between Outcome and Process Supervision
06:08 Impact of Active Learning
07:09 Conclusion and Future Research Directions
💡 VIDEO SUMMARY 💡
This video provides a detailed analysis of the "Step by Step" research paper from OpenAI. The authors explore how large language models perform complex multi-step reasoning tasks and discuss how these models can be made more reliable.
Two key methods are compared in the study: outcome supervision and process supervision. The former provides feedback on the final result, while the latter provides feedback for each intermediate reasoning step. The research demonstrates that process supervision significantly outperforms outcome supervision when tested on a challenging math dataset.
The authors also discuss how active learning enhances the efficiency of process supervision, and introduce PRM 800k, a dataset of 800,000 step-level human feedback labels used for training their best reward model.
Furthermore, the authors tackle the problem of false positives in AI reasoning, highlighting instances where the model generates errors that escape detection. The video discusses these issues in detail and underscores the importance of careful supervision in training these models.
💼 RELATED WORK 💼
The video also references related studies, including research by Wei Sato et al. 2022 and GAO et al. 2022, that contribute to the understanding of outcome and process supervision, and reinforcement learning from human feedback respectively.
🔮 FUTURE RESEARCH 🔮
The authors encourage future research to focus on improving the diversity of data for active learning and investigating the iterative retraining process's impact. They also underline the importance of developing methods to handle false positives and enhancing the overall reliability of large language models.
-
2:05:49
Badlands Media
1 day agoDevolution Power Hour Ep. 336
91.6K33 -
14:11
DeVory Darkins
14 hours ago $26.11 earnedFetterman SLAMS Democrats during shocking MSNBC Interview
72.7K80 -
8:42
Chris Williamson
1 year agoThe Harsh Reality Of Our Collapsing Birthrate - Jordan Peterson
34.8K19 -
4:33:31
Alex Zedra
11 hours agoLIVE! Playing Split Ficition!
61.3K7 -
2:51:38
TimcastIRL
12 hours agoGovernment SHUTDOWN IMMINENT, Democrats Vow To BLOCK Trump CR w/The Native Patriot | Timcast IRL
197K103 -
3:38:57
Digital Social Hour
1 day ago $21.06 earnedAndrew Tate EXPOSES the Truth About Legal Battles, Politics & Masculinity | Andrew Tate DSH #1231
75K22 -
2:26:29
Laura Loomer
12 hours agoEP108: Dems Embrace Domestic Terrorism To "Get Trump"
72.9K36 -
3:01:51
Right Side Broadcasting Network
14 hours agoWATCH: NASA’s SpaceX Crew-10 Launch
135K46 -
2:06:17
Glenn Greenwald
14 hours agoJudge Orders Hearing on Columbia Student Deportation Case; Is the Ukraine Ceasefire Plan Serious? Trump Attacks Thomas Massie for His Budget Vote | SYSTEM UPDATE #422
142K203 -
47:16
BonginoReport
16 hours agoTrump-Elon Bromance Triggers The Libs (Ep.03) - 03/12/2025
171K313