Premium Only Content
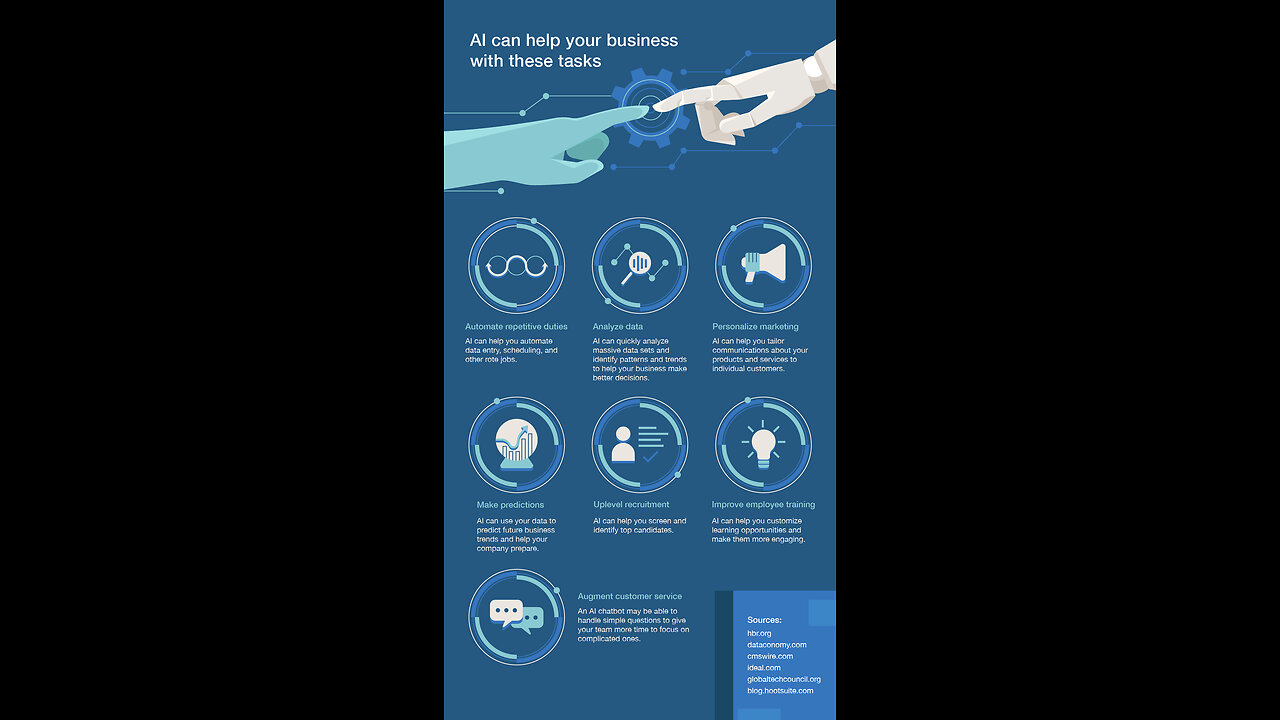
How to Train Generative AI Using Your Company's Data - HBR.org Daily
🥇 Bonuses, Promotions, and the Best Online Casino Reviews you can trust: https://bit.ly/BigFunCasinoGame
How to Train Generative AI Using Your Company's Data - HBR.org Daily
Leveraging a company’s proprietary knowledge is critical to its ability to compete and innovate, especially in today’s volatile environment. Organizational innovation is fueled through effective and agile creation, management, application, recombination, and deployment of knowledge assets and know-how. However, knowledge within organizations is typically generated and captured across various sources and forms, including individual minds, processes, policies, reports, operational transactions, discussion boards, and online chats and meetings. As such, a company’s comprehensive knowledge is often unaccounted for and difficult to organize and deploy where needed in an effective or efficient way. Many companies are experimenting with ChatGPT and other large language or image models. They have generally found them to be astounding in terms of their ability to express complex ideas in articulate language. However, most users realize that these systems are primarily trained on internet-based information and can’t respond to prompts or questions regarding proprietary content or knowledge. Leveraging a company’s propriety knowledge is critical to its ability to compete and innovate, especially in today’s volatile environment. Organizational Innovation is fueled through effective and agile creation, management, application, recombination, and deployment of knowledge assets and know-how. However, knowledge within organizations is typically generated and captured across various sources and forms, including individual minds, processes, policies, reports, operational transactions, discussion boards, and online chats and meetings. As such, a company’s comprehensive knowledge is often unaccounted for and difficult to organize and deploy where needed in an effective or efficient way. Emerging technologies in the form of large language and image generative AI models offer new opportunities for knowledge management, thereby enhancing company performance, learning, and innovation capabilities. For example, in a study conducted in a Fortune 500 provider of business process software, a generative AI-based system for customer support led to increased productivity of customer support agents and improved retention, while leading to higher positive feedback on the part of customers. The system also expedited the learning and skill development of novice agents. Like that company, a growing number of organizations are attempting to leverage the language processing skills and general reasoning abilities of large language models (LLMs) to capture and provide broad internal (or customer) access to their own intellectual capital. They are using it for such purposes as informing their customer-facing employees on company policy and product/service recommendations, solving customer service problems, or capturing employees’ knowledge before they depart the organization. These objectives were also present during the heyday of the “knowledge management” movement in the 1990s and early 2000s, but most companies found the technology of the time inadequate for the task. Today, however, generative AI is rekindling the possibility of capturing and disseminating important knowledge throughout an organization and beyond its walls. As one manager using generative AI for this purpose put it, “I feel like a jetpack just came into my life.” Despite current advances, some of the same factors that made knowledge management difficult in the past are still present. The Technology for Generative AI-Based Knowledge Management The technology to incorporate an organization’s specific domain knowledge into an LLM is evolving rapidly. At the moment there are three primary approaches to incorporating proprietary content into a generative model. Training an LLM from Scratch One approach is to create and train one’s own domain-specific model from scratch. That’s not a common approach, since it requires a massive amount of high-quality data to train a large language model, and most companies simply don’t have it. It also requires access to considerable computing power and well-trained data science talent. One company that has employed this approach is Bloomberg, which recently announced that it had created BloombergGPT for finance-specific content and a natural-language interface with its data termina...
-
12:14
DeVory Darkins
6 hours ago $15.53 earnedBill Maher TRUTH BOMB Triggers HEATED Argument on The View
23K42 -
31:58
The Officer Tatum
3 hours agoThe View LASHES OUT On Morning Joe For MEETING WITH Trump
15.7K25 -
1:37:46
Kim Iversen
9 hours agoJoe Biden’s Post-Election Revenge: WW3 | Democrats Tremble Over Matt Gaetz and RFK Jr, Form “Shadow Cabinet"
100K116 -
1:47:09
Fresh and Fit
8 hours agoMoney Monday Call-In Show
64K9 -
1:48:50
Glenn Greenwald
9 hours agoDC Attacks Trump's Most Disruptive Picks; Biden Authorizes Massive Escalation With Russia; Joe & Mika Meet With "Hitler" | SYSTEM UPDATE #367
152K145 -
1:44:34
Tucker Carlson
8 hours agoTucker Carlson and Russ Vought Break Down DOGE and All of Trump’s Cabinet Picks So Far
193K245 -
1:42:47
Flyover Conservatives
1 day agoBO POLNY | The Best and Worst Times Are Coming – Are You Ready? | FOC Show
44.1K17 -
51:12
BIG NEM
11 hours agoWelcome to Our Uncensored Show: Trump, Simulation Theory & the Albanian Mob - EP1
60.5K16 -
2:05:14
Robert Gouveia
10 hours agoFBI Criminals Get LAWYERS; STOP Counting ILLEGAL Votes; Time to Disbar Tish James
72K106 -
1:00:30
The StoneZONE with Roger Stone
8 hours agoAre We Heading For World War III? General Michael Flynn Joins The StoneZONE w/ Roger Stone
50.5K13