Premium Only Content
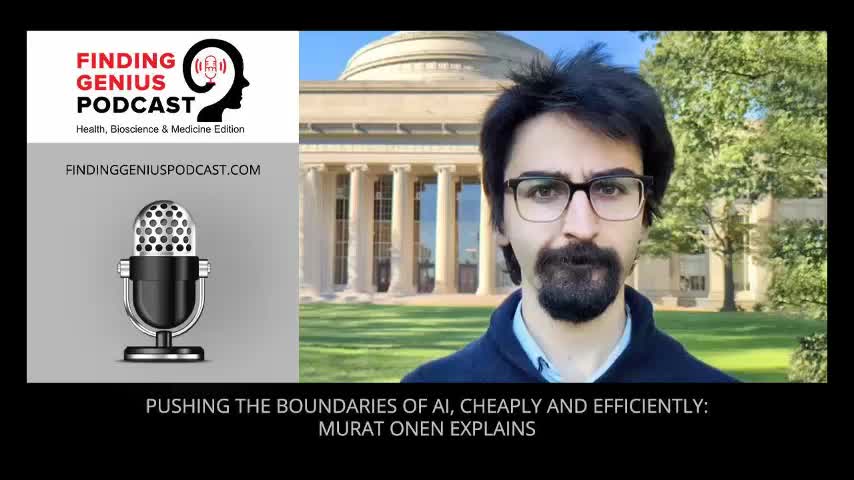
Pushing the Boundaries of AI, Cheaply and Efficiently: Murat Onen Explains
Large-scale AI models that enable next-generation applications like natural language processing and autonomous systems require intensive training and immense power. The monetary and environmental expense is too great.
This is where analog deep learning comes into play. The concept behind it is to develop a new type of hardware that can accelerate the training of neural networks, achieving a cheaper, more efficient, and more sustainable way to move forward with AI applications.
Murat Onen, a postdoctoral researcher in the Department of Electrical Engineering and Computer Science at MIT, explains.
Tune in to explore:
Conventional vs. novel methods of training neural networks
The difference between GPUs and CPUs and why it matters
Analog vs. digital machine operations
About how long it will take to have small and full-scale systems that outperform conventional AI models
Press play for the full conversation.
-
0:34
FGP
1 day ago🌱 Gerson Therapy: The Controversial Cancer Cure 🌱
251 -
1:35:54
Glenn Greenwald
12 hours agoBiden Welcomes "Hitler" Back To The White House; Trump's Latest Appointments: What Do They Mean?; Jeremy Loffredo On Imprisonment In Israel | SYSTEM UPDATE #365
156K158 -
1:41:41
The Officer Tatum
13 hours agoLIVE: Joe Biden MEETS With "Hitler" as Media CRIES Over Pete Hegseth Becoming Sec of Defense
126K134 -
1:42:59
vivafrei
12 hours agoMAKING AMERICA GREAT AGAIN! Trump's Cabinet Picks Are Making Democrats GO CRAZY!!! Viva Frei Live!
154K69 -
54:56
LFA TV
1 day agoThe Trump Resurgence and Leadership Chaos in Germany | Trumpet Daily 11.13.24 9PM EST
90.2K11 -
55:51
PMG
1 day ago $9.41 earned"Uncovering the Nature of God and Faith!"
67.7K3 -
15:09
Cooking with Gruel
8 hours agoLive in the kitchen!
59.6K -
1:00:40
The StoneZONE with Roger Stone
9 hours agoPresident Trump Makes Triumphant Return to Washington D.C. | The StoneZONE
77K14 -
1:55:27
Tundra Gaming Live
11 hours ago $3.96 earned🛑 Former F-16 Maintainer (Weapons) Plays War Thunder//F-16C and Gamblers Skin Grind🛑
46.2K -
1:40:25
Megyn Kelly
12 hours agoElon and Vivek Prepare For DOGE, Pete Hegseth to Pentagon, and Trump Goes to DC, with Bill Ackman
90.6K69